Understanding AI Agents: The Future of Intelligent Task Management
- info058715
- Apr 21
- 5 min read
AI agents represent a new wave of digital assistants designed to autonomously handle a wide range of tasks that traditionally required human intervention. Whether assisting with natural language processing, automating workflows, or making complex decisions, these intelligent software entities are reshaping how people and organizations engage with technology.
What Exactly Is an AI Agent?
An AI agent is a software-based system that can independently make decisions, take action, and adapt to its environment to accomplish goals. Unlike traditional automation tools, AI agents collaborate, learn from experience, and function in dynamic, real-world settings.
We’re already familiar with early forms of AI agents, like customer service chatbots or generative AI tools for writing and coding. But modern agents are far more capable. They can coordinate with other agents, adjust based on feedback, and tackle increasingly sophisticated assignments across different domains.
With major tech players such as Google, Microsoft, and OpenAI developing infrastructure to support AI agent ecosystems, we are witnessing a shift from passive digital tools to proactive problem solvers. Platforms like Microsoft Copilot, Amazon Q, and the upcoming Project Astra indicate a future where AI agents are as fundamental to productivity as mobile apps are today.
Categories of AI Agents
AI agents come in a variety of forms depending on their purpose. Below are some of the most common categories:
Personal Productivity Assistants (Copilots): These agents are tailored to support individual users by generating content, answering questions, or automating repetitive tasks. Think of them as hyper-efficient digital teammates.
Task Orchestrators: These agents manage and automate workflows or entire business processes. They often integrate with existing tools, coordinating multiple steps to deliver outcomes more efficiently.
Domain-Specific Solutions: Designed for particular business functions—such as IT operations, finance, or customer service—these agents rethink task execution within their niche areas.
AI-Driven Business Models: Some organizations are embedding agents across their operations, transforming how products and services are delivered without just optimizing existing methods.
AI-Based Virtual Workers: These agents act like digital employees, fulfilling tasks in traditional business frameworks and helping scale capacity without hiring more human labor.
Many organizations implement a hybrid approach—deploying productivity assistants for individual tasks while testing more advanced agents in operational workflows.
How AI Agents Operate
AI agents typically operate in four core phases:
Instruction: The user provides a prompt, question, or problem for the agent to solve.
Planning & Delegation: The agent breaks the task into smaller parts and assigns them to specialized sub-agents if necessary.
Iteration & Feedback: The system gathers input, adjusts, and refines the response as needed.
Execution: The agent completes the task—whether generating a report, sending an email, or executing a workflow.
They operate across both human-facing interfaces (like websites or dashboards) and machine-level environments (such as APIs), allowing them to perform complex actions across digital systems.
Collaboration and Self-Improvement
One of the most powerful aspects of modern AI agents is their ability to collaborate—with humans and with each other. Agents can pass tasks between one another, evaluate peer outputs, and even engage in internal feedback loops to enhance performance.
For example, one agent might generate content while another reviews it for accuracy, clarity, or bias. These feedback mechanisms help agents learn, refine results, and expand their skill sets over time.
Ethical AI frameworks can also be embedded within agent architectures. For instance, a secondary agent may be tasked with reviewing outputs to ensure they meet organizational standards or legal compliance.
AI Agents and Language Models
While not all AI agents rely on large language models (LLMs), many of those designed for communication or content creation do. LLMs excel at interpreting and generating human language, making them especially useful in applications like digital assistants, marketing bots, and virtual support agents.
In more specialized use cases—such as image recognition or predictive analytics—AI agents may rely on other forms of machine learning models, each tailored to its task.
Business Impact and Opportunities
AI agents present significant opportunities for productivity, innovation, and cost reduction. By streamlining workflows, modernizing legacy systems, and enabling agile operations, AI agents are becoming foundational to digital transformation strategies.
Companies already adopting AI agents are reporting improvements in software development timelines, customer service metrics, and internal efficiency. Over time, these agents may take on increasingly autonomous roles, managing operations with minimal human oversight and driving continuous optimization.
Potential Use Cases
AI agents have broad applications across industries. A few promising examples include:
Financial Services: Agents can evaluate creditworthiness, assess loan risks, and automate parts of the underwriting and approval process.
Legacy IT Overhaul: AI agents are being used to modernize outdated codebases, migrate old systems, and document processes for compliance and maintainability.
Marketing Automation: From campaign planning and execution to real-time performance optimization, networks of agents can deliver end-to-end marketing solutions.
These are just early signs of what’s possible. As AI agents continue to evolve, entire business units may be powered by self-operating systems of coordinated agents.
Key Challenges to Adoption
Despite their potential, AI agents face several obstacles:
Trust and Reliability: Many users are still skeptical of AI systems, especially in high-stakes or customer-facing roles. Building trust requires transparency, consistency, and accountability in agent performance.
Change Management: Deploying AI agents isn’t just a software upgrade—it requires rethinking workflows, reskilling employees, and adapting team dynamics.
Data Governance: As agents often process sensitive or proprietary data, it’s essential to implement strong governance practices, including security protocols, audit trails, and compliance monitoring.
Transforming Technology Infrastructure
AI agents are also influencing how organizations structure their digital ecosystems. Instead of relying solely on monolithic software platforms, businesses may begin to use networks of lightweight, collaborative agents. Three potential models include:
Integrated Platforms: These provide prebuilt systems with embedded agents that can communicate across business tools without extensive customization.
AI Middleware (Wrappers): These secure interfaces allow agents to interact with enterprise systems while shielding proprietary data.
Custom AI Agents: Built in-house, these agents are trained on private datasets and customized to perform niche tasks within a company’s operations.
This modular approach allows businesses to scale more flexibly and respond to changing needs quickly.
How to Get Started
For organizations ready to explore AI agents, here are three steps to consider:
Audit Major Initiatives: Look at large projects where time or budget overruns are common. These areas may be ripe for AI-driven improvements.
Target High-Impact Problems: Focus your agent deployment on processes that are critical to your operations and stand to benefit most from automation or optimization.
Prepare for Change: Build internal readiness by investing in training, updating tech stacks, and creating a roadmap that aligns AI agent deployment with strategic goals.
Final Thoughts: Understanding AI Agents
Understanding AI Agents. AI agents aren’t just another trend in the tech landscape—they represent a transformative shift in how digital work gets done. As the tools mature and organizations adapt, these intelligent systems will reshape operations, workflows, and job roles across industries.
From individual productivity boosters to enterprise-wide orchestration engines, AI agents are rapidly becoming the backbone of the future workplace.
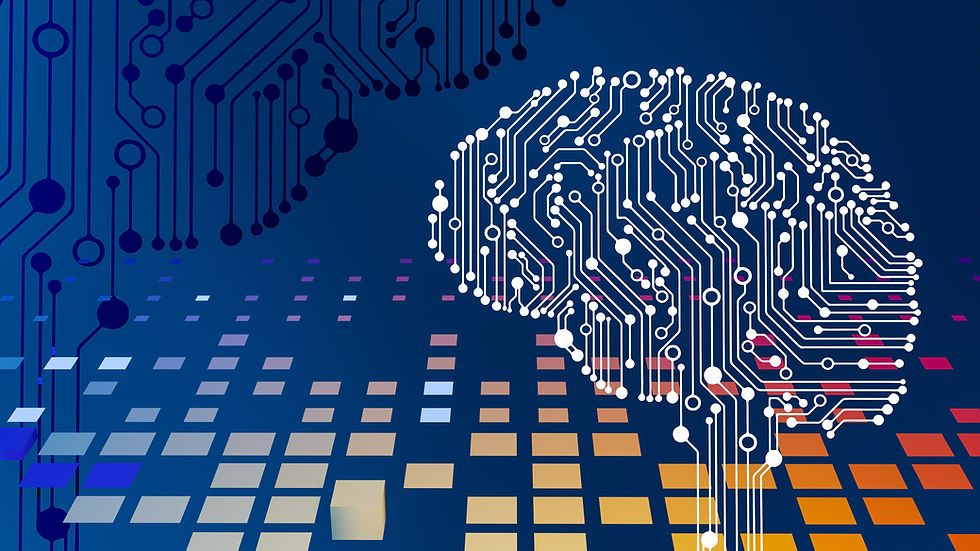
Comments